Data Virtualization for Azure with
a powerful combination of Lyftrondata
Microsoft Azure is a public cloud computing platform that offers a range of services, including analytics, storage, and networking. It’s extensible, allowing users to select and integrate the services that work for their specific needs. Azure compatibility with open source technologies gives users the option to select the tools they prefer. It offers four different forms of cloud computing: infrastructure as a service (IaaS), platform as a service (PaaS), software as a service (SaaS) and serverless.
Lyftrondata has created Azure’s ultimate data virtualization architecture—a real-time replication and federation system that delivers superior agility, speed, and responsiveness.
Build Azure synapse data warehouse securely and swiftly
Operational efficiency while virtualizing data on Azure
To facilitate the rapid change of data, a virtualized model is faster than a physical model. When implementing data virtualization in Azure, you can avoid data loss if you use views to virtualize data as it moves amid different zones. Physical objects can be recovered up to a point before an error with Azure’s Time Travel. Lyftrondata’s virtualization helps in eliminating the error & resuming the process from exactly where it was hampered. Extensively virtualized data impacts Azure’s Zero Copy Cloning. This enables users to clone the metadata of schemas, tables, or the whole database and copy it. If an individual clone can’t be copied, a schema or a database can be cloned that consists of views if the underlying tables that provided the data for those specific views were cloned.
How could the performance of Azure be impacted in a virtualized model
Performance is an essential factor for any analytics platform. If performance is too slow, data analysts won’t be able to gather the information they need quickly. Different platforms perform differently, so it’s important to understand how Azure performs vs. other systems. By physically creating some objects and virtualizing others, performance can be boosted—Azure stores data in its proprietary structure known as a micro-partition. Partition Pruning determines how fast Azure can process queries based on statistics gathered by the system while storing information.
Azure employs a range of techniques to use the most pertinent partitions for any given query and identify those partitions that don’t contain data relevant to the question. More specifically, Azure clusters data in the view and optimizes queries that reference the view by first identifying which partitions are relevant to the query and then determining how much of the clustered dataset those partitions contain.
Enterprise grade data replication for Azure SQL data
Understanding the cost impact of a highly virtualized design
Azure is inexpensive in terms of the physical storage required. However, you must consider all computational costs when dealing with a highly virtualized design. If Azure stores your data in its warehouse and it cannot fit the entire dataset into memory, then when it processes your query, it spills the data to disk to work. This will degrade your query performance. To avoid this from happening, Azure recommends that you modify the query predicates to increase partition pruning, reducing the amount of data it has to process. This will minimize or eliminate spilling to the disk during its computation process. You can also increase Azure’s warehouse-size for this purpose.
Every query involves the transformation processing of a highly virtualized model. This is negligible in situations where views are used sparingly, but the cost increases for substantially nested scenarios.
Data Virtualization for Azure with a powerful combination of Lyftrondata
When you combine the Lyftrondata data virtualization platform with Azure, you can get all of the benefits of Azure’s native scalability, speed, and flexibility, along with the data virtualization capabilities that are, in some cases, hard to find when you work with other data virtualization software. This article will explain how well the Lyftrondata data virtualization platform and Azure augment each other to enable a flexible, scalable data architecture.
Data Virtualization with Lyftrondata empowers users to integrate data from disparate sources, provides greater flexibility in data access, limit data silos, and automates query execution for faster time-to-insight. Leverage data virtualization to complement processes like data warehousing, data preparation, data quality management, and data integration.
Lyftrondata Data Virtualization makes it easy for business users and professional IT developers to access data from Azure. Lyftrondata Data Virtualization delivers database server independence and hides the SQL dialect of the data source in use. Azure’s data storage service is accessed via SQL. Customers can use other APIs or languages depending on the requirements of their data-storage consumers. Views that join several sources are processed more efficiently with Lyftrondata data virtualization, allowing metadata, view, and tag. Business users and professional IT developers can learn which views are dependent on which sources via a search-based metadata system.
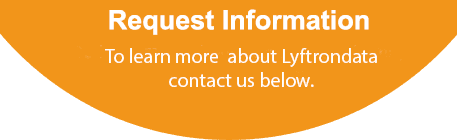
Are you unsure about the best option for setting up your data infrastructure?
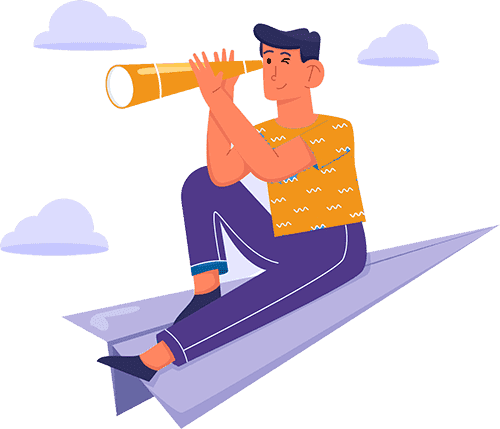