Data Virtualization for Google Bigquery with a powerful combination of Lyftrondata
Data Virtualization with Google BigQuery
BigQuery’s fully managed cloud data warehouse lets you use SQL to analyze terabytes and petabytes of data. Imagine how easier it is to manage your business when you can answer all your organization’s biggest questions with built-in analytics and take action on your insights in real-time. BigQuery’s serverless architecture and scalable, distributed analysis engine make it easy to get real results quickly – no infrastructure management required.
Google BigQuery ML lets you easily build and scale machine learning (ML) models with the power of BigQuery. This documentation covers all aspects of BigQuery ML, including installation, model management and deployment, training models, and API reference.
Enterprise grade data platform for Google BigQuery
Data virtualization architecture of Google BigQuery
BigQuery’s serverless architecture allows us to offer you predictable and a low cost of use. The compute resources needed to run your queries are only spun up when you need them and reclaimed when they’re idle. This allows us to offer cheap query prices and scale our service up or down as needed, which in turn means we can pass the savings on to you!
A data virtualization architecture creates a layer of technology that acts as an abstraction layer between the user and the one or more data stacks needed in an enterprise. The key concept in virtualization is creating schematic models of the data from various types of sources within an enterprise. The purpose is to create schematics that can be used to plan for reporting requirements and to understand what information is available before selecting reports for use.
How the performance of Google BigQuery could be impacted in a virtualized model?
Performance is an important factor for any analytics platform. You need to understand how you can improve the performance of Google BigQuery. You should consider using a virtualized model rather than a physical model to improve performance. You can boost performance by improving the design, using less virtualized objects, and making sure your queries are run on powerful machines.
Data meets data virtualization in modern data architecture
Data Virtualization for Google BigQuery with a Powerful Combination of Lyftrondata
Data virtualization, when combined with Lyftrondata, lets you access your data in an easy way. You can use Google BigQuery as a source for cached views. Data virtualization might otherwise seem superfluous when used with Google BigQuery, but if you consider the whole data architecture responsible for data storage, processing, and analytics, you will clearly understand how well the Lyftrondata data virtualization platform and Google BigQuery augment each other to enable a flexible, scalable data architecture.
Lyftrondata data virtualization integrates many kinds of data and allows it to be queried as one database. Out of that database, one can query data sources like Google BigQuery. Lyftrondata Data Virtualization also lets you access data from different sources, including spreadsheets, SQL databases, and even other services. This technology essentially helps with the inherent heterogeneity of current data processing systems.
Stakeholders can consolidate security across disparate data sources. Lyftrondata virtualization eliminates the need for different security specifications for various data sources by handling all security specifications in one uniform way.
Moreover, Data Virtualization hides the SQL dialect of each data source in use. It provides independence to the database server and defines all integrations, aggregations, filtering, and transformation specifications using views. Lyftrondata Data Virtualization offers consumers API or language-neutral access to data stored in Google BigQuery.
Lyftrondata’s data virtualization solutions allow users to execute complex queries, including distributed joins, without having to get the data into a central system. By defining views and their associated columns, users can search metadata and discover views depending upon the sources.
Moreover, lineage can be used to know what adjustments have been established overtime on the particular data. Thus, data lineage can also be utilized to trace the source of the information used for a view, and this will also give insight into how changes could impact a different view. This can be done through impact analysis and people can understand the consequences of modifications before they are actually implemented.
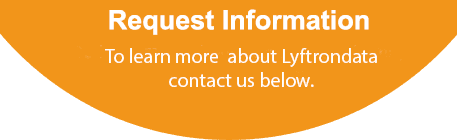
Are you unsure about the best option for setting up your data infrastructure?
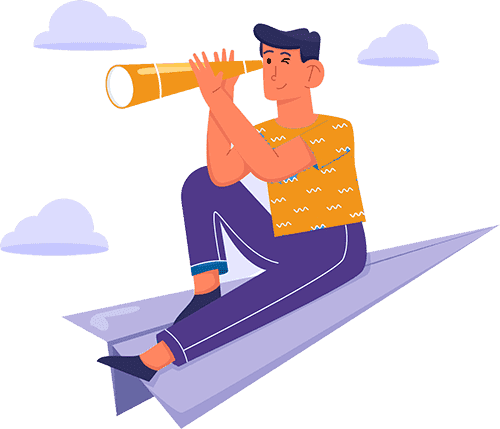